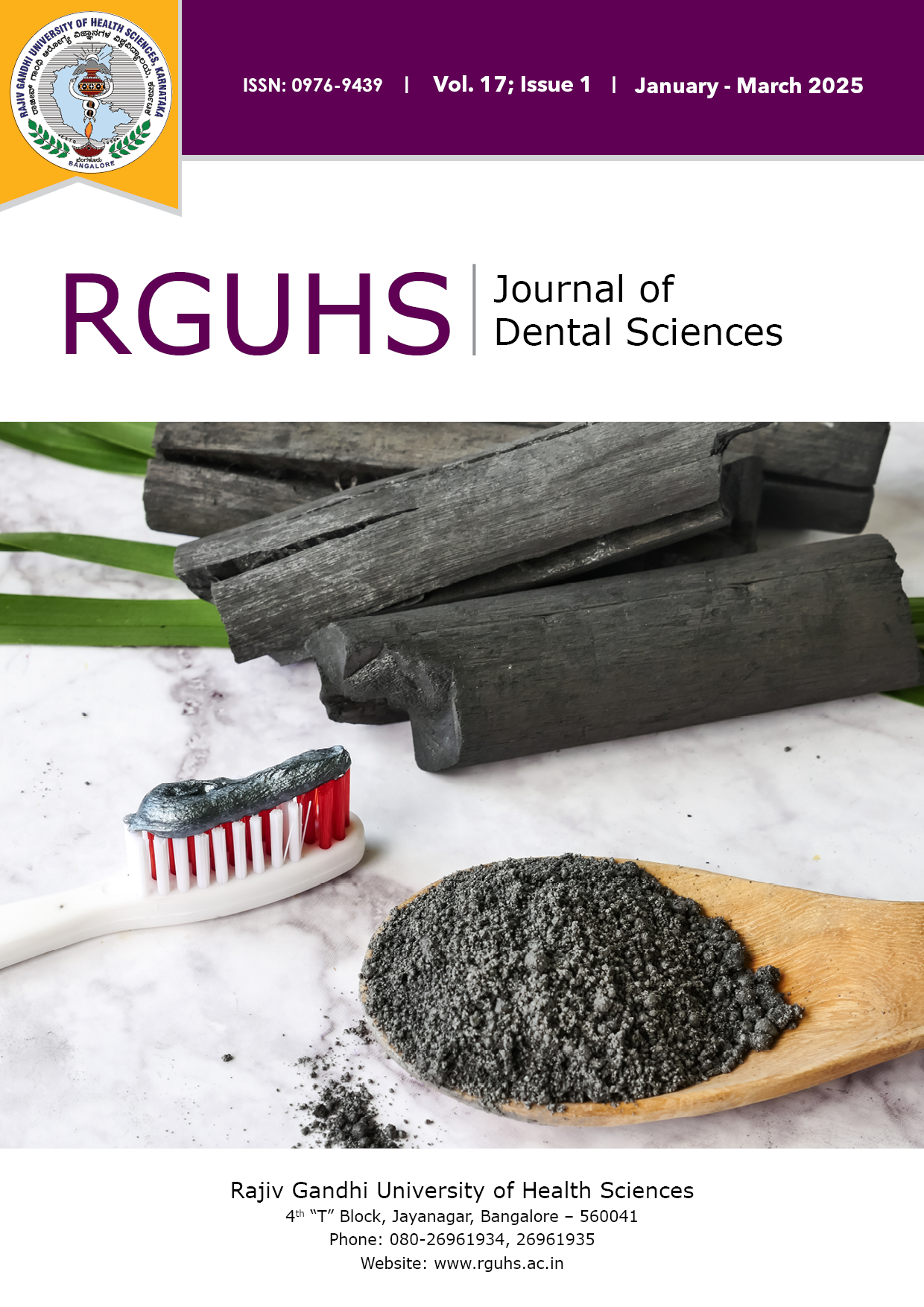
RGUHS Nat. J. Pub. Heal. Sci Vol No: 17 Issue No: 1 pISSN:
Dear Authors,
We invite you to watch this comprehensive video guide on the process of submitting your article online. This video will provide you with step-by-step instructions to ensure a smooth and successful submission.
Thank you for your attention and cooperation.
1Dr. Seema Shantilal Pendharkar, Associate Professor (Reader), Department of Oral and Maxillofacial Surgery, CSMSS Dental College and Hospital, Chhatrapati Sambhajinagar, Maharashtra, India.
2Department of Oral and Maxillofacial Surgery, CSMSS Dental College and Hospital, Chhatrapati Sambhajinagar, Maharashtra, India
*Corresponding Author:
Dr. Seema Shantilal Pendharkar, Associate Professor (Reader), Department of Oral and Maxillofacial Surgery, CSMSS Dental College and Hospital, Chhatrapati Sambhajinagar, Maharashtra, India., Email: dr.seemapendharkar@gmail.com
Abstract
With its roots in the information technology industry, artificial intelligence is a revolutionary force powered by complex software mechanisms that offer multiple advantages in a broad range of societal domains. Among these, its integration with the dental field in the healthcare industry has shown to be a significant success. Artificial intelligence (AI) is a multifaceted health technology instrument that is transforming healthcare by applying personalized, proactive, interactive and anticipatory methods. AI comprises various computing ideas, including neural networks, machine learning, and deep learning methods. AI presents a multitude of options for improving preoperative planning, intraoperative workflow, and postoperative patient outcomes in the field of oral and maxillofacial surgery (OMFS). This review was conducted to analyse the present uses of AI in oral and maxillofacial surgery (OMFS) and to equip surgeons with the necessary technical elements to comprehend the potential offered by this technology.
Keywords
Downloads
-
1FullTextPDF
Article
Introduction
The field of Artificial Intelligence (AI) is one that is developing quickly. It uses large datasets and computer science to improve problem-solving skills. AI technologies provide a multitude of advantages to people in their daily lives by imitating human intelligence. AI has been smoothly incorporated into cultures all over the world, from the removal of spam emails to the development of wearables that can distinguish between aerobic and regular activities using accelerometer sensors and personalized product recommendations from online retailers.1,2
The application of AI in oral and maxillofacial surgery (OMFS) offers the promise of improved surgical planning, precision, and patient outcomes. With the analysis of complicated medical imaging data, including computed tomography (CT) scans and three-dimensional (3D) models, artificial intelligence (AI) systems can help with preoperative assessments, virtual surgical simulations, and the creation of personalized treatment regimens. Sophisticated algorithms and machine learning approaches are used to achieve this. Additionally, by improving surgical precision, lowering complications, offering real-time advice and feedback, AI can help doctors make decisions during surgery.3,4
AI has the potential to enhance preventative dentistry by facilitating early diagnosis and better treatment outcomes. Fast data processing, archiving and comparisons are made possible by software and AI integration.5 With the ultimate goal of strengthening the role of dental surgeons, the technology seeks to reduce errors in dental procedures, optimize workflows, and assist clinical decision-making.6 The objective of the current narrative review article was to investigate the ways in which AI is applied to dentistry and related fields, as well as the ways in which AI addresses human proficiency levels in various dental situations.
Applications of AI in Oral and Maxillofacial Surgery
By bringing cutting-edge instruments and techniques that dramatically improve the precision, effectiveness, and results of surgical treatments for the reconstruction and rehabilitation of maxillofacial abnormalities, artificial intelligence (AI) is poised to revolutionize the field of oral and maxillofacial surgery (OMFS). Important uses of AI in OMFS include:
1. Computer-Aided Design and Computer-Aided Manufacturing (CAD/CAM)
CAD/CAM uses digital imaging, 3D photography, intraoral scans and 3D printing to create personalized guides, plates, implants and prostheses that are made to fit the unique anatomy of the maxillofacial deformities that need to be repaired. This technique could lower expenses, expedite the reconstruction process and enhance the devices' fit, functionality and appearance.7
2. Machine Learning (ML) and Deep Learning (DL)
Complex datasets and patterns are analyzed using ML and DL algorithms, which facilitates more precise decision-making across OMFS domains.8 AI models built on ML and DL have proven to be highly effective in tasks like localizing anatomical components like the inferior alveolar nerve and making decisions about tooth extractions.9
3. Artificial Neural Networks (ANNs)
ANNs are used in OMFS to help with analysis and decision-making by modelling intricate relationships and processes.10 AI has been specifically used to make decisions about tooth extractions using cone-beam computed tomography (CBCT) and panoramic pictures, demonstrating how it can help with treatment planning.11
4. Bioprinting and Tissue Engineering
AI makes it easier to use bioprinters to create scaffolds that can hold tissue engineering products and grafts, providing creative alternatives for maxillofacial defect reconstruction. This strategy has the potential to produce tailored, biocompatible structures that aid in the regeneration of tissues in the oral and maxillofacial area.
These programs work together to increase precision, economy and general standard of care for patients undergoing maxillofacial reconstruction by incor-porating AI into OMFS. In addition to improving the surgical procedure, technology could result in better patient satisfaction and postoperative results.12
Convolutional neural networks, which enable the identification and categorization of characteristics on panoramic radiographs and cone-beam CT images, are widely used in oral surgery.13 These tumorous or cystic lesions, which are frequently odontogenic in origin, present a diagnostic and treatment difficulty. Their clinical histories are typically similar, their radio-graphic findings are frequently polymorphic and they lack any pathognomonic signs of a specific diagnosis. A more aggressive approach may be necessary for certain lesions, including ameloblastomas, to reduce the risk of tissue loss, malignant transformation, and recurrence, whereas other lesions only require restricted surgical care of enucleation and curettage.14
One of the most frequent procedures carried out by oral, maxillofacial and dental surgeons is the extraction of impacted third molars. To optimize the several phases of management, algorithms have been put forth. A prediction model of the eruption potential of the third molars through automatic determination of their angulation on panoramic radiographs can help with the sometimes contentious decision regarding the surgical recommendation for tooth extraction.15 Even though complications are still common, particularly neurological ones, certain instruments can help direct the process by anticipating surgical difficulty or by identifying a contact with the inferior alveolar canal on panoramic radiographs.16,17
AI has so far been used in implantology to identify the type of implant on panoramic radiographs, forecast the success of osteointegration or the survival of implants using various input data and enhance implant design by maximizing the porosity, length and diameter of implants to reduce stress at the implant-bone interface.18
AI has also been applied to robotic surgery in the OMFS domain. Successful outcomes have been shown for AI-assisted cranial surgical techniques, like temporomandibular joint surgery, dental implants, tumour removal and biopsies.19 Research indicates that as compared to conventional freehand techniques, AI-assisted surgery increased oral implant treatments' accuracy and safety. In particular, the application of AI lessened the necessity in certain instances for revision procedures and implant repositioning.20 Moreover, AI-assisted methods decreased the requirement for extra treatments by enabling more accurate surgical removal of cysts and tumors.21
Uses of AI in Oral and Maxillofacial Surgery
The application of this innovative technology in orthognathic surgeries can aid patients with dentofacial deformities (DFD) throughout the orthodontic-surgical care process, from initial diagnosis to postoperative follow-up. The bulk of studies address cephalometric diagnosis support and automatic teleradiograph annotation tools. Redistributing medical time, improving measurement repeatability, and ensuring that results are comparable are all made possible by automating this labour-intensive operation that has been demonstrated to have considerable inter-operator variability. Nowadays, algorithms are thought to be at least as efficient as experts, and a wide range of products are being developed. Numerous research works have suggested 3-D image analysis instruments to enhance the depiction of specific intricate DFD attributes. In comparison to other computational techniques previously used, machine learning enables the quick and reliable detection and interpretation of the numerous bone and skin landmarks needed for a thorough 3D analysis. For patients with DFD, the surgeon and orthodontist must have experience doing irreversible surgery. Treatment decision support tools that anticipate the need for surgery during orthodontic treatment can currently be produced by training algorithms on cephalometric data, unannotated imaging studies or photographs. This kind of algorithm assists the practitioner in verifying or re-evaluating his choice to reduce the use of abusive surgical procedures that are costly and intrusive, or orthodontic camouflage that occasionally yields unfavourable functional and aesthetic effects.22-24
AI models have demonstrated exceptional perfor-mance, closely emulating the accuracy and precision of dental specialists with training. Panoramic radiographs for clinical conditions like age estimation, osteoporosis, vertical root fractures, automatic teeth detection and numbering, apical lesions, maxillary sinusitis, detecting and segmenting the approximate location of the inferior alveolar nerve and mandibular third molar, periodontal bone loss, gender determination and temporomandibular joint osteoarthritis have all been read by AI algorithms as a result of numerous studies. While some studies examine the link between third molar (M3) and inferior alveolar nerve (IAN), they often assess whether AI is capable of identifying M3 and IAN on CBCT or panoramic radiographs, which are easily observable to humans. A recent study found that individuals could readily discern the level of complexity of extraction by deep learning.25,26
Conclusion
The process of making decisions in surgical practice is intricate and might involve the practitioner's values and emotions. This can result in cognitive bias that could negatively influence the treatment of patients. Despite this, these factors have only recently begun to be studied in depth.
In the field of maxillofacial surgery, artificial intelli-gence can support practitioners in their day-to-day work by offering tools to aid in diagnosis, therapeutic decision-making, preoperative planning, and surgical outcome prediction. These new technologies benefit almost every area of the specialty. In fact, in certain activities, they are already outperforming professionals. Nonetheless, certain obstacles remain, especially with regard to ethics and data security, before patients can receive effective, reliable and repeatable enhanced medicine. Maxillofacial surgeons will need to grasp these new instruments and participate in their development and implementation within the medical system. Introducing these newer technologies to younger generations and methodically incorporating data into clinical practice will be essential for their successful adoption.
In the years to come, collaborative databases and decompartmentalization of engineering and medical courses will likely facilitate the successful introduction of AI into the field, overcoming current challenges.
In order to enhance understanding of AI models and algorithms, residency programs at OMFS are recommended to include fundamental AI courses in their training offerings. Program directors have the option to engage specialists in healthcare AI to deliver basic and clinical level courses. Residents will be able to critically assess models for their application and securely use AI technology into their practice. To enhance upcoming AI models, OMFS residents must to be motivated to provide high-calibre, prospective research while they are still in training. When AI becomes a routine treatment, the field can seamlessly switch to using AI by building models on future databases that are more predictable. OMFS surgeons working together internationally can generate high-quality AI training data from a range of patient demographics, ensuring their continued importance in the development and implementation of AI in the maxillofacial area.
There are a gazillion possible uses for AI in OMFS. AI can make it easier to identify illnesses, anomalies, and fractures during diagnostic procedures. Prognostic indicators from high-quality database models can be very helpful in surgery planning and post-operative care. For example, developing a model to determine the probability of implant failure through the analysis of imaging data and the consideration of medical comorbidities, drugs, and other relevant risk factors could revolutionize the field of implantology.
Conflicts of Interest
Nil
Supporting File
References
1. Limketkai BN, Mauldin K, Manitius N, et al. The age of artificial intelligence: use of digital tech-nology in clinical nutrition. Curr Surg Rep 2021;9(7):20.
2. Clarke SL, Parmesar K, Saleem MA, et al. Future of machine learning in paediatrics. Arch Dis Child 2022;107:223-228.
3. Rokhshad R, Keyhan SO, Yousefi P. Artificial intelligence applications and ethical challenges in oral and maxillo-facial cosmetic surgery: A narrative review. Maxillofac Plast Reconstr Surg 2023;45(1):14.
4. Rasteau S, Ernenwein D, Savoldelli C, et al. Artificial intelligence for oral and maxillo-facial surgery: A narrative review. J Stomatol Oral Maxillofac Surg 2022;123(3):276-282.
5. Tandon D, Rajawat J, Banerjee M. Present and future of artificial intelligence in dentistry. J Oral Biol Craniofac Res 2020;10(4):391-396.
6. Aminoshariae A, Kulild J, Nagendrababu V. Artificial intelligence in endodontics: current applications and future directions. J Endod 2021;47(9):1352-1357.
7. Chang SW, Abdul-Kareem S, Merican AF, et al. Oral cancer prognosis based on clinicopathologic and genomic markers using a hybrid of feature selection and machine learning methods. BMC Bioinformatics 2013;14(1):1-15.
8. Scrobotă I, Băciuț G, Filip AG, et al. Application of fuzzy logic in oral cancer risk assessment. Iran J Public Health 2017;46(5):612-619.
9. Pereira KR, Sinha R. Welcome the “new kid on the block” into the family: artificial intelligence in oral and maxillofacial surgery. Bri J Oral Maxillofac Surg 2020;58:83-84.
10. McCullough M, Ly S, Auslander A, et al. Convo-lutional neural network models for automatic preoperative severity assessment in unilateral cleft lip. Plast Reconstr Surg 2021;148(1):162-169.
11. Spoer DL, Kiene JM, Dekker PK, et al. A systematic review of artificial intelligence applications in plastic surgery: Looking to the future. Plast Reconstr Surg Glob Open 2022;10(12):e4608.
12. Rasteau S, Ernenwein D, Savoldelli C, et al. Artificial intelligence for oral and maxillo-facial surgery: A narrative review. J Stomatol Oral Maxillofac Surg 2022;123(3):276-282.
13. Orhan K, Bayrakdar IS, Ezhov M, et al. Evaluation of artificial intelligence for detecting periapical pathosis on cone-beam computed tomography scans. Int Endod J 2020;53(5):680-9.
14. Effiom OA, Ogundana OM, Akinshipo AO, et al. Ameloblastoma: Current etiopathological concepts and management. Oral Dis 2018;24:307-16.
15. Vranckx M, Van Gerven A, Willems H, et al. Artificial intelligence (Ai)-driven molar angulation measurements to predict third molar eruption on panoramic radiographs. Int J Environ Res Public Health 2020;17(10):3716.
16. Yoo JH, Yeom HG, Shin W, et al. Deep learning based prediction of extraction difficulty for mandibular third molars. Sci Rep 2021;11(1):1954.
17. Fukuda M, Ariji Y, Kise Y, et al. Comparison of 3 deep learning neural networks for classifying the relationship between the mandibular third molar and the mandibular canal on panoramic radiographs. Oral Surg Oral Med Oral Pathol Oral Radiol 2020;130(3):336-43.
18. Revilla-León M, Gómez-Polo M, Vyas S, et al. Artificial intelligence applications in implant dentistry: A systematic review. J Prosthet Dent 2023;129(2):293-300.
19. Knoedler L, Baecher H, Kauke-Navarro M, et al. Towards a reliable and rapid automated grading system in facial palsy patients: Facial palsy surgery meets computer science. J Clin Med 2022;11(17):4998.
20. Jaemsuwan S, Arunjaroensuk S, Kaboosaya B, et al. Comparison of the accuracy of implant position among freehand implant placement, static and dynamic computer-assisted implant surgery in fully edentulous patients: A non-randomized prospective study. Int J Oral Maxillofac Surg 2023;52(2):264-271.
21. Balaban C, Inam W, Kennedy R, et al. The Future of dentistry: How AI is transforming dental practices. Compend Contin Educ Dent 2021;42(1):14-17.
22. Bouletreau P, Makaremi M, Ibrahim B, et al. Artificial Intelligence: Applications in orthog-nathic surgery. J Stomatol Oral Maxillofac Surg 2019;120(4):347-354.
23. Schwendicke F, Chaurasia A, Arsiwala L, et al. Deep learning for cephalometric landmark detection: systematic review and meta-analysis. Clin Oral Investig 2021;25(7):4299-309.
24. Hwang HW, Park JH, Moon JH, et al. Automated identification of cephalometric landmarks: Part 2-Might it be better than human? Angle Orthod 2019;90(1):69-76.
25. Jaskari J, Sahlsten J, Jarnstedt J, et al. Deep learning method for mandibular canal segmen-tation in dental cone beam computed tomography volumes. Sci Rep 2020;2020(10):5842.
26. Kwak GH, Kwak EJ, Song JM, et al. Automatic mandibular canal detection using a deep convolu-tional neural network. Sci Rep 2020;10(1):5711.